Shadow AI Security in SaaS: Risks, Challenges, and How to Protect Data

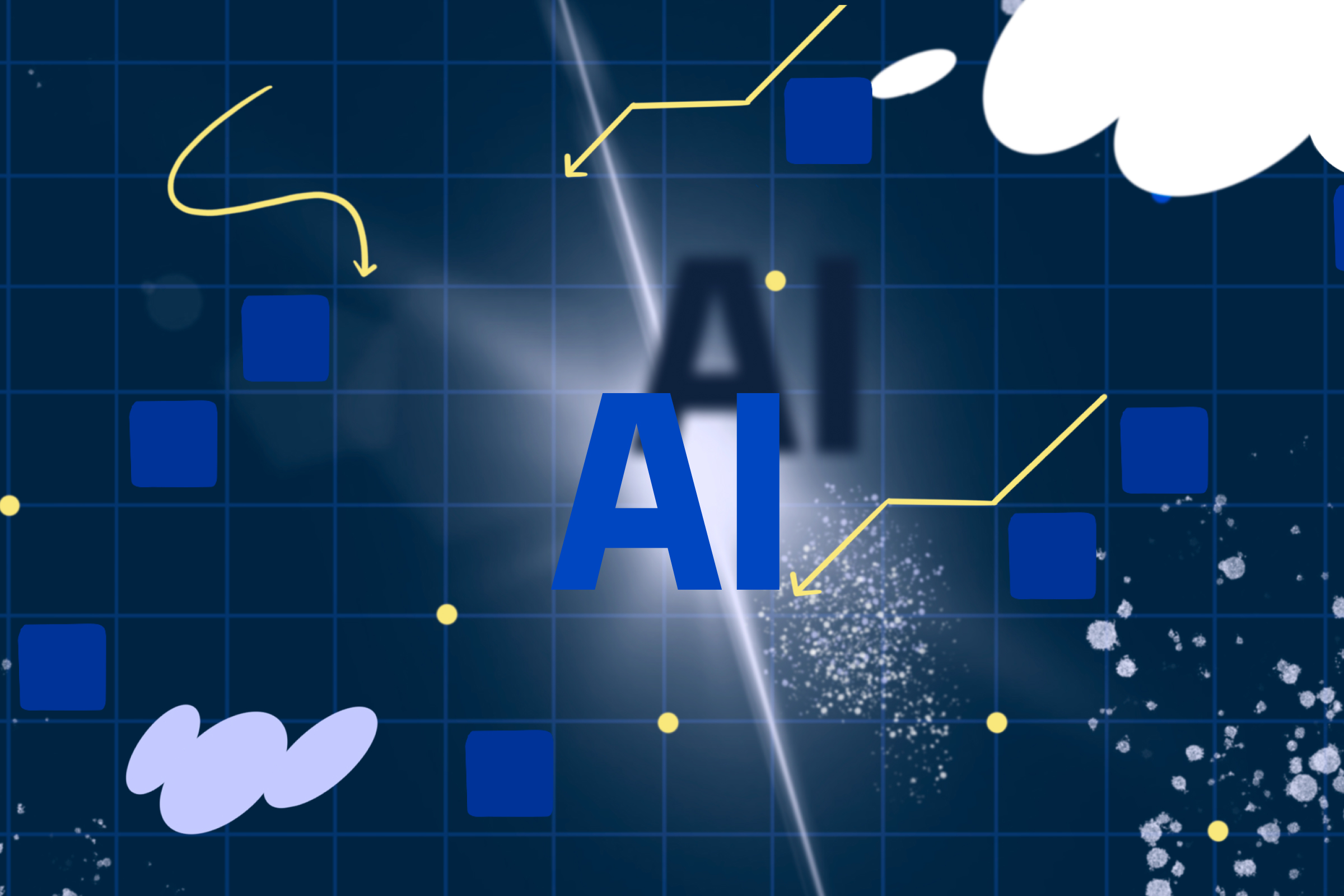
The recent surge in AI adoption has sparked a race amongst companies to integrate AI into their products and services, seeking to deliver more value to users. That’s good news for efficiency-seeking employees, but unfortunately it's given rise to a new security concern: shadow AI. A subset of shadow IT, shadow AI refers to the unapproved or unsanctioned use of AI tools and copilots within an organization. While AI can boost efficiency and innovation, when left unregulated, it can create serious security risks.
Types of Shadow AI
Shadow AI tools can be categorized into three types based on how they interact with systems.
Standalone Shadow AI
Standalone shadow AI applications are AI tools that are being used by employees to support business functions, but they’re not integrated with corporate infrastructure. For example, a developer uses a ChatGPT instance to assist with writing code or a marketing manager uses Jasper to generate content. While these applications may seem harmless at the surface, there’s still the chance that employees will expose private data by inputting company information into these tools. In fact, recent studies indicate that 15% of employees regularly post company data into AI tools, with over a quarter of that data being sensitive information.
Integrated Shadow AI
Shadow AI applications that are connected to the organization's approved systems are much more dangerous. Through APIs or other integration points, these tools may exchange information with approved applications or share access across multiple platforms. This broadens the attack surface area as threat actors can potentially use these shadow AI platforms as a gateway to access other SaaS applications.
Shadow AI Copilots
AI copilots are AI assistants that are embedded within applications and designed to streamline tasks and increase employee productivity. From GenAI to Agentic AI, they can help analyze data, generate content, or automate processes within a given app. Because shadow AI copilots are often built into approved business applications, they can be even more tricky for Security teams to discover and secure.
Shadow AI Security Risks
Like traditional shadow applications, shadow AI applications run the risk of becoming compromised, but they also create unique risks due to their ability to ingest and share information.
Data Exposure
The most severe risk stems from permanent data exposure through model sharing and training. When employees input sensitive information into consumer AI tools, that data becomes irretrievable as it enters the AI makers' black box of training models. Recent studies show that 38% of employees share sensitive work information without permission, and over 80% of legal documents are exposed through non-corporate AI accounts.
Increased Risk of breach
Shadow AI increases the attack surface area by introducing unapproved and unmonitored AI tools into an organization's environment. These tools often integrate with other applications via APIs or OAuth connections, creating new entry points for attackers. Without IT oversight, they may lack proper security configurations, increasing the risk of exploitation or lateral movement within the network.
Unauthorized Access
AI operators gain persistent access to corporate information through human review of customer prompts and training data. This risk intensifies when employees use personal accounts that lack enterprise security protections, creating a natural security degradation compared to corporate identity providers.
Permanent Data Exposure
Once employees begin uploading data to AI systems, that information can never be fully recalled or erased. This permanence creates a unique challenge where detection must focus on prevention rather than remediation, as traditional data recovery methods prove ineffective.
Misinformation
AI tools are constantly learning from users, the internet, and from training data. While this increases their ability to provide value, naturally, there is a risk that AI models will train on false information and then produce inaccurate information and recommendations for users. When productivity-seeking employees do not fact check the information generated, there’s a risk they will utilize that bad information in corporate communications, from marketing to customer interactions. This can create mistrust in the brand, or worse, produce suboptimal outcomes for customers and business partners.
Supply Chain Attacks
Introducing AI tools that integrate with sanctioned business tools create new attack vectors that bad actors can exploit to gain access to sensitive data or infiltrate connected applications. Once compromised, attackers can move laterally across the organization’s supply chain, impacting multiple vendors and partners. This was seen in the Snowflake attack, where an insecure Snowflake environment was used to break into customers like Ticketmaster, Santander Group, and AT&T.
Why Traditional Discovery Tools Fall Short
Traditional tools were built before the era of GenAI. Here's why they don't sufficiently detect shadow AI.
CASB
CASB tools and secure web gateways can log SaaS app traffic by monitoring network connections. They often maintain a catalog of known SaaS services (often tens of thousands of apps) and can recognize when users access those services. This can be useful for spotting some unsanctioned apps. However, if an app isn’t in the CASB’s catalog, it may go unnoticed. New startups or AI tools might not be recognized until the CASB vendor updates their databases.
Additionally, CASBs rely on traffic going through corporate networks or devices – with today’s remote work and mobile access, employees might use shadow apps off the VPN or on personal devices, bypassing those monitors.
Since CASBs look at network traffic, they can't understand anything about the data being entered into a service. So they might log that a user visited an AI website, but they won't know if sensitive data was entered, making them unable to accurately rank risks.
Finally, since CASBs are identifying apps by domain names and IP addresses, they can't detect embedded AI copilots or agents that may share a domain with approved apps.
DLP
DLP solutions scan for sensitive data leaving the organization (through email, file transfers, etc.) and can sometimes detect patterns (like credit card numbers or personal data) being uploaded to web forms. In theory, a DLP could catch someone trying to paste a social security number into an external web app. However, in practice, DLP is hard to fine-tune – too strict, and it blocks legitimate work; too lax, and it misses incidents. A lot of shadow IT interactions slip past DLP because they may not trigger obvious policy violations.
For instance, if an employee uses an AI assistant and types a product roadmap description into it, that might not match a specific DLP pattern, yet its sensitive intellectual property being shared. DLP also doesn’t tell you which new app the data went to – it might just alert “possible data exposure” without the full context, causing Security professionals to invest a lot of time to figure out what's going on. And like CASB, if the usage happens from an unmanaged device or encrypted channel, DLP might not see it at all.
Browser Extensions
Browser extension SaaS security tools promise to monitor user activity, prevent data leakage, and secure your SaaS environment with minimal effort. But the problem is, these extensions end up introducing more security risks than they solve. Browser extensions require extensive permissions:
- Access to browsing history
- Ability to read and modify web page content
- Monitor keystrokes and form submissions
- Access to cookies and authentication tokens
These permissions create new security vulnerabilities:
- New Attack Surface: Even trusted extensions from reputable vendors can be hijacked and replaced with malicious versions, as demonstrated by the Cyberhaven supply chain attack.
- Increased Risk Exposure: Extensions that monitor credentials create a single point of failure—if compromised, attackers gain access to login credentials across all sites
- Privacy Concerns: Extensions with broad permissions can potentially expose sensitive company and customer data, raising compliance issues
Plus, significant coverage gaps make browser extensions ineffective:
- Multi-Browser Reality: Extensions only work in specific browsers, missing activity on other browsers (Safari, Firefox, Brave, etc.)
- Mobile Blind Spots: Extensions don't work on mobile devices, where increasingly more SaaS access occurs
- Deployment Challenges: Achieving 100% deployment across all employee devices is practically impossible, creating security blind spots
How Reco Discovers and Secures Shadow AI
Reco’s Dynamic SaaS Security Platform was built with AI in mind to help you discover and manage shadow AI and SaaS, as well as embedded Copilots and Agents. We employ Email Header Metadata Scanning technology in order to discover apps in use and provide insight into usage. We can also flag potentially risky data uploads.
Some providers scan the whole email (header and body), which is a potential security issue because those tools may ingest sensitive business info. Reco's technology is lightweight and minimally invasive. By scanning the email header looking for signs of registration, usage, and uploads, it can answer questions like:
- Which shadow AI tools are in use in my environment?
- Who is using them and when?
- What actions have these users taken? Any potentially risky uploads?
- What permissions do these usershave and how are they authenticating?
- Are these shadow AI tools connected to any other SaaS tools in my environment?
→ Learn More About How Reco Works, Read the Blog: Uncovering Shadow Apps and Shadow AI with Reco
To learn more about how to protect your business from shadow AI download The CISOs Guide to Shadow AI. Or reach out to Reco to schedule a demo to learn how Reco can help you manage and mitigate shadow AI risk.

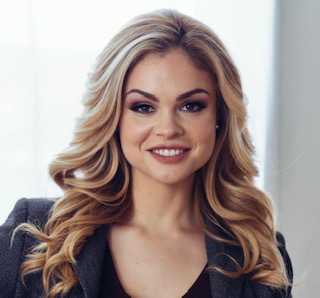