GenAI Security: Risks, Benefits and Best Practices
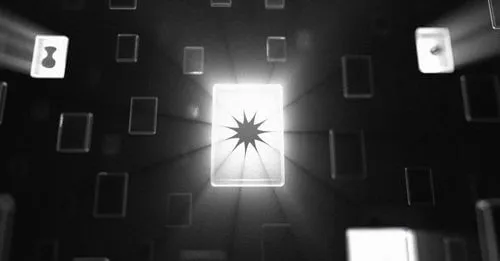
What is GenAI Security?
GenAI Security refers to the practices, technologies, and policies implemented to mitigate the risks associated with generative AI systems. It focuses on ensuring the safety of large language models (LLMs) and preventing data exposure, malicious manipulations like prompt injections, and unintended or harmful outputs. These measures aim to maintain secure operations and trustworthy results in generative AI applications.
Key Pillars of GenAI Security
Effective GenAI Security relies on foundational principles that address the unique risks posed by generative AI technologies. These pillars ensure the safety, reliability, and compliance of systems throughout their lifecycle by integrating technical measures and industry best practices:
- Data Privacy and Encryption: Sensitive data used in generative AI must be encrypted both at rest and in transit using industry-standard protocols like AES-256. Advanced techniques such as homomorphic encryption enable computations on encrypted data, further reducing the risk of exposure. Organizations must also adopt secure key management practices to prevent unauthorized decryption.
- Robust Authentication Measures: Multi-factor authentication (MFA) and role-based access control (RBAC) are essential to restrict access to generative AI systems. Zero Trust architectures further enhance security by continuously validating user identity and device trust before granting access to sensitive AI resources.
- Model Protection and Integrity: AI models are at risk of adversarial manipulation, such as tampering with weights or injecting malicious data during training. Techniques like adversarial training, digital signatures, and hash verification ensure model integrity by preventing unauthorized modifications. Regular audits of model performance help identify deviations caused by adversarial inputs.
- Input Validation and Sanitization: To defend against prompt injection attacks, all user inputs must be validated and sanitized before being processed by the model. Implement techniques like input filtering, tokenization, and escaping of special characters to minimize the risk of malicious inputs bypassing security protocols.
- Continuous Monitoring and Threat Detection: Deploy real-time monitoring systems to track usage patterns, detect anomalies, and respond to threats. AI-driven security tools can identify unusual behaviors, such as repeated attempts to extract sensitive data, and alert security teams for immediate action.
- Trusted Supply Chain: Use datasets and pre-trained models from verified, reputable sources to avoid risks associated with unverified or compromised inputs. Establish a process for vetting third-party integrations, plugins, and APIs to prevent supply chain attacks that could infiltrate your AI systems.
- Compliance and Ethical AI Practices: Ensure generative AI systems comply with data protection regulations such as GDPR, HIPAA, or CCPA. Implement automated compliance checks to monitor adherence to these frameworks. Ethical considerations, such as bias detection and transparency, are equally critical to maintaining trust in AI outputs.
- Secure Deployment Strategies: Generative AI systems must be deployed in controlled environments. Use techniques such as network isolation, container orchestration (e.g., Kubernetes), and advanced firewall configurations to limit exposure to external threats. Regularly update deployment environments to address emerging vulnerabilities.
Risks Associated with GenAI
While generative AI offers tremendous potential, it also introduces unique risks of Generative AI in SaaS platforms that can compromise data security, operational integrity, and organizational trust. These risks can be categorized into three primary areas: Usage Risks, Integration Risks, and Model-Specific Risks, each requiring specific attention to mitigate their impact.
Examples of Security Breaches in AI Systems
AI systems have made groundbreaking advancements, but their implementation has not been without flaws. Two notable incidents that follow highlight the real-world consequences of security lapses and unintended outcomes in AI technologies.
Tesla's Autopilot Malfunction
In 2020, Tesla faced scrutiny due to malfunctions in its Autopilot system. The National Highway Traffic Safety Administration (NHTSA) conducted a two-year investigation into a series of crashes linked to Autopilot, leading to a recall of over 2 million vehicles in the U.S. The recall aimed to address a defect in the system designed to ensure drivers remain attentive while using Autopilot. Tesla issued a software update to rectify the issue.
Amazon's AI Recruitment Tool Bias
In 2018, Amazon discontinued an AI-driven recruitment tool after discovering it exhibited bias against female candidates. Developed to streamline the hiring process, the tool was trained on resumes submitted over a decade, which were predominantly from male applicants. Consequently, the AI system learned to favor male candidates, leading to discriminatory hiring recommendations. Recognizing the inherent bias, Amazon scrapped the project to prevent potential discriminatory practices.
Benefits of GenAI Security
Securing generative AI systems while mitigating risks also unlocks significant advantages for organizations. From strengthening data protection to ensuring compliance, the benefits of GenAI Security are transformative.
- Enhanced Visibility Into AI Operations: Provides security teams with deeper insights into how AI models function and are applied, enabling proactive risk identification and management.
- Protection Against Data Breaches: Protects sensitive information by preventing unauthorized access or exposure during data processing or model outputs.
- Improved Compliance With Regulations: Ensures alignment with data protection laws, such as GDPR, by incorporating policies that uphold privacy and transparency in AI operations.
- Reduction in AI Misuse: Prevents harmful applications of generative AI, such as the creation of malicious content or manipulation of outputs, through strict access controls and monitoring.
- Mitigation of Financial Risks From Security Breaches: Reduces the financial impact of potential security incidents by minimizing risks that could lead to costly breaches or reputational damage.
How to Mitigate GenAI Risks: Best Practices
Effectively managing the risks associated with generative AI requires a proactive and strategic approach. The following best practices provide a foundation for ensuring the secure and responsible use of these advanced systems:
- Implement Access Controls: Restrict access to generative AI systems and data by enforcing strict identity verification and permissions management.
- Monitor User Behavior: Track user activities to detect unusual patterns or potential misuse of generative AI tools.
- Manage Data Exposure: Limit the use of sensitive data in prompts and ensure proper encryption during storage and transmission.
- Detect Shadow AI Usage: Monitor and address the presence of unauthorized AI tools, including generative AI, within the organization. Often referred to as shadow AI, these unapproved tools can bypass established security controls, increasing the risk of data exposure and compliance violations.
- Ensure Compliance: Align generative AI practices with relevant legal and regulatory standards to avoid penalties and maintain trust.
- Conduct Security Audits: Regularly evaluate systems to identify and address security gaps or weaknesses.
- Establish Incident Response Plans: Develop and test protocols to handle security breaches or AI misuse effectively and minimize impact.
- Educate Stakeholders: Provide ongoing training to employees and stakeholders on the secure use and risks of generative AI technologies.
GenAI Security with Reco
As organizations increasingly adopt generative AI tools, securing the SaaS environments where these tools operate is essential. Reco empowers security teams with comprehensive visibility, actionable insights, and proactive measures to mitigate risks associated with generative AI while ensuring compliance with organizational policies.
- Enhanced Visibility: Reco maps SaaS ecosystems, uncovering all applications, users, and their interactions to provide a comprehensive view of the organization’s security posture. This includes the detection of shadow AI tools that may be operating without IT approval, reducing risks tied to unauthorized AI usage.
- Generative AI Discovery: Reco’s advanced Generative AI discovery feature identifies and monitors generative AI applications across SaaS environments. It ensures these tools are securely deployed, reducing risks of misuse or data leakage.
- Data Risk Management: By monitoring sensitive data interactions, Reco identifies risks such as unauthorized sharing or accidental exposure. Its capabilities align with the principles of SaaS security, offering actionable insights to prevent further incidents and maintain compliance.
- Compliance Monitoring: Reco ensures compliance with regulatory frameworks like GDPR and HIPAA, providing detailed audit trails and real-time monitoring of SaaS applications.
Conclusion
Generative AI offers immense potential, but its adoption comes with unique security challenges that demand proactive management. By implementing the best practices and addressing key risks, organizations can unlock the benefits of generative AI while ensuring its safe and responsible use. With the right strategies, generative AI can become a transformative tool without compromising security or trust.
If you're seeking to enhance the security of your SaaS applications and gain comprehensive visibility into every app and identity, Reco offers an AI-based platform designed to integrate seamlessly via API within minutes. Book a demo today to see how Reco can help secure your SaaS ecosystem with ease.